Tech you can trust: Crafting ethical AI experiences in UX design

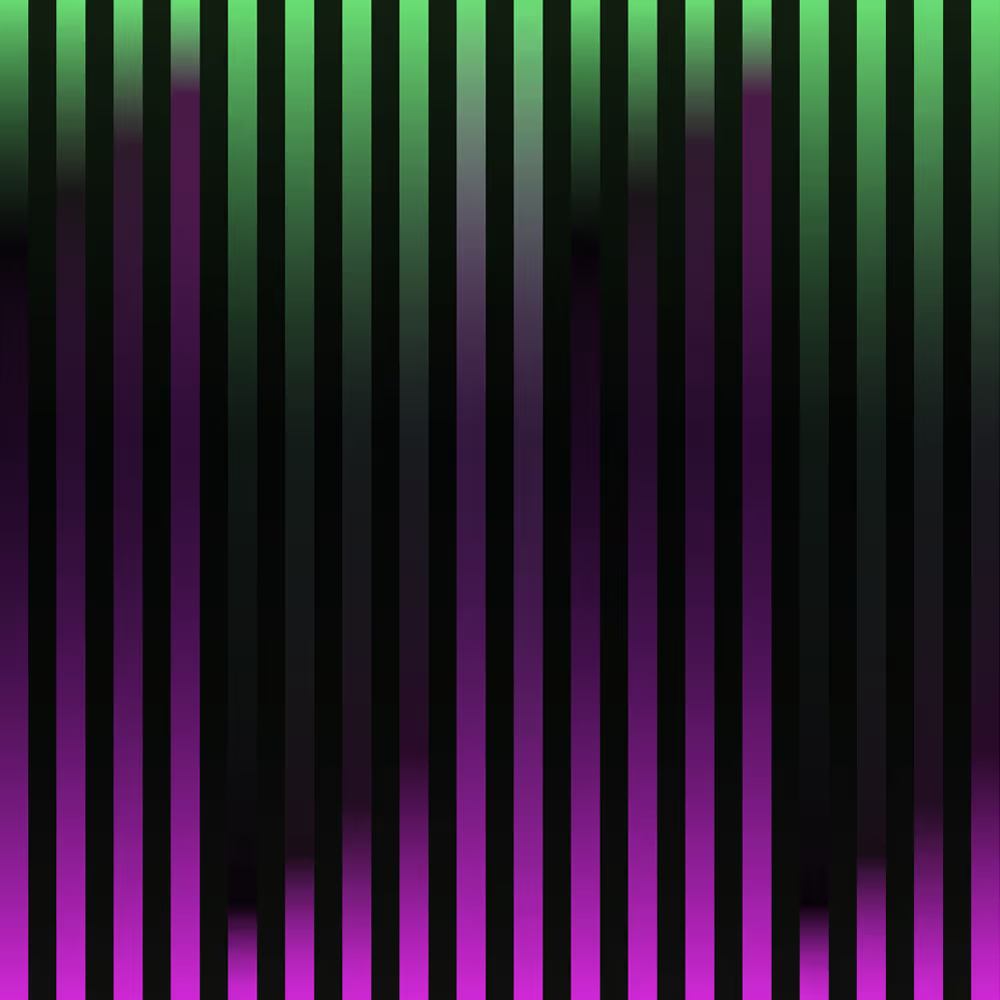
Artificial Intelligence (AI) is playing an increasingly important role in our daily lives, blurring the boundaries between technology and human connection. As we integrate this technology into everything from our workflows, to our cars, to our healthcare and beyond, our ever-growing interest in this technology has provoked thoughtful conversations about the complexities of AI's ability to mimic genuine interactions, the ethical considerations surrounding data privacy, and the right balance between man and machine.
This week, Launch by NTT Data General Manager and professor of UX Design Lisa Woodley joins Chris and Gina to dissect the top ethical considerations surrounding AI. Check out the highlights below, then dive into the full episode to learn more about how the next generation of designers are approaching technology ethics.
The role of the designer
How do you keep the human touch in the age of AI? You entrust your user experience (UX) design to humans committed to upholding both quality and ethical standards. Designers should act as the ‘guardians of the human,’ questioning decisions and acting as advocates for the user’s best interests. To ensure this focus is maintained, involve designers early in AI projects.
Client conversations & FOMO
No one wants to be the one left at home on a Friday night while all their friends are at the big party. In the same way, clients often express a fear of missing out on opportunities that their competitors may be seizing. They don’t want to be the ones lagging behind while others race ahead, and they’re eager to adopt AI to gain an edge. Most clients want to adopt AI for cost savings and top-line growth, but there’s often an underlying sense of urgency that stems from a desire to keep up. To help avoid shiny object syndrome and the potential of adopting AI that will do more harm than good, clients should be given clear guidance on how to contextualize and apply AI solutions based on their specific business problems, not based on what everyone else is doing.
Responsibility to disclose
Let’s face it. We’ve all asked ourselves, “Am I talking to a real person?” when using a live chat feature. To take the guesswork out of it and help avoid potential ethical failures, there should be clear disclosure when users are interacting with AI, especially in emotionally sensitive situations.
Skepticism and faith in technology
Approximately 30% of the population has never known life without the internet, while a further 25% gained access to technology early on in their lives. Naturally, these younger generations are more comfortable with and therefore may have more faith in technology due to having grown up with it, while older generations might approach it with more skepticism. Either way, designers should never leave their users to question whether the AI system they’re using is trustworthy. Transparency and insight into the decision-making processes of AI systems is critical to fostering greater trust among users.
Complexity of data and bias
AI is not inherently biased, but bias can creep in through the data it is trained on. Unfortunately, a complete prevention of biased data is neither feasible nor achievable. Instead, we must make our efforts to mitigate bias in AI decision-making more thorough with a clear data governance process in place to identify when something is going wrong.
Design thinking approach
If you don’t know why you’re adopting AI in the first place, you probably shouldn’t be doing it at all. Any AI implementation should start by identifying client problems that need to be solved, and then thoughtfully applying AI based on those specific business needs. AI should fit the problem, not the other way around.
As always, don’t forget to subscribe to Catalyst wherever you get your podcasts. We release a new episode every Tuesday, and each one is jam-packed with expert advice and actionable insights for creating digital experiences that move millions.