Breaking down silos: Edge AI with Shahid Ahmed
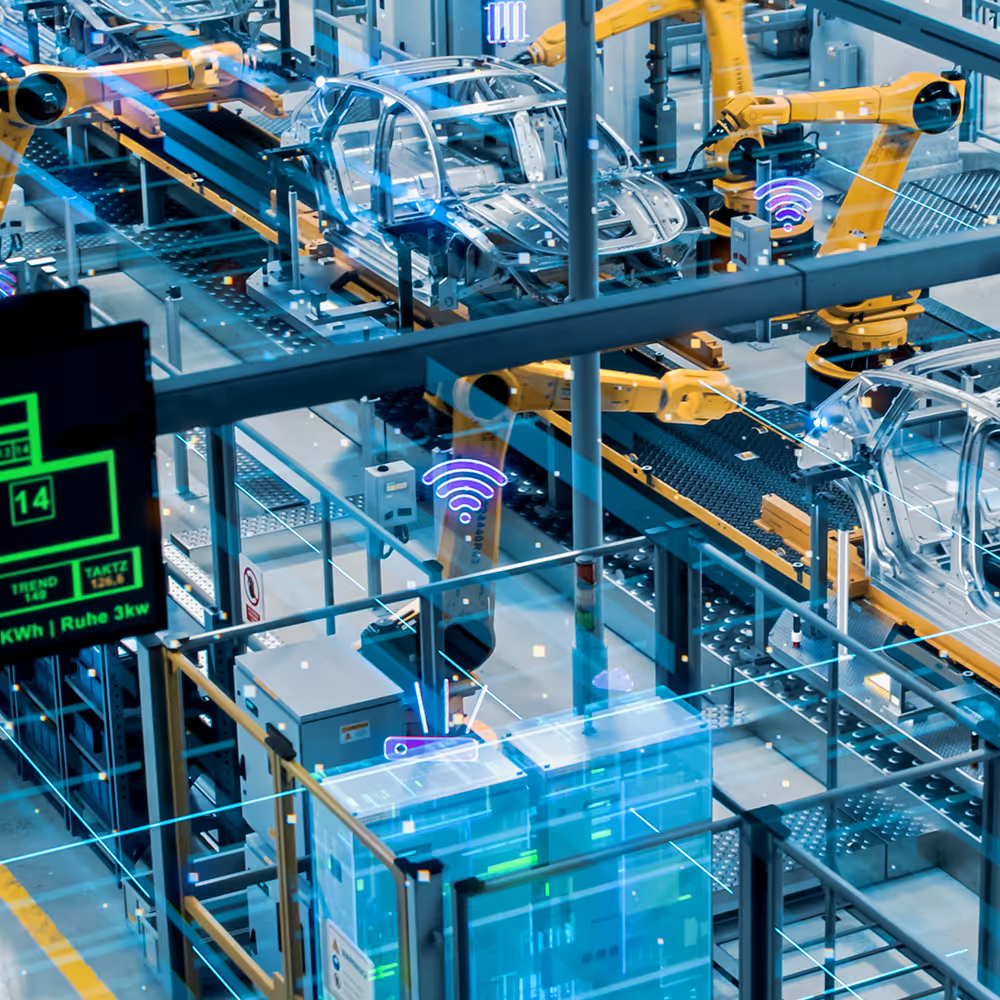
Everyone wants to be on the cutting edge, but when it comes to AI, certain businesses are lagging behind their peers on adoption and implementation. On this episode of Catalyst, Clinton is joined by Shahid Ahmed, Group EVP at NTT DATA, to discuss how NTT DATA’s new Edge AI solution is being used to improve efficiency and operations in environments such as factories, hospitals, and beyond.
On the cutting edge
Over the past several years, we’ve integrated AI into our everyday lives and watched some truly remarkable use cases take shape among enterprises. But outside of what Shahid considers “the IT world” in places like shipyards, airports, hospitals, and factories, adoption has been more of a challenge. NTT DATA recently introduced an Edge AI solution to help solve for this.
We’ve become very familiar with GenAI and its capabilities thanks to tools like ChatGPT, Copilot, and the like. These LLMs are static, heuristic based, statistical probabilistic models. Edge AI is actionable and dynamic. It takes real time action on the data that it is receiving and continuously learns, with the whole AI lifecycle running locally on a small compute footprint. This is ideal for non-IT world environments wherein conditions can change at the drop of a hat.
Keeping data under control
As with any form of AI, considerations must be made regarding data handling. This need is heightened in places like hospitals or factories where data is especially sensitive or mission critical.
Helping to power Edge AI is Private 5G (P5G) which secures the data and ensure it stays local. This is critically important for security and is also necessary for Edge AI to be most effective. In physical scenarios where Edge AI is deployed, it must be able to compute in as near real time as possible. Latency to and from the cloud erodes effectiveness, it simply takes too long to compute and put a decision into action. Conversely, compute at the edge, secured by P5G, and charged with Edge AI brings and keeps real-time decision making local.
You can imagine an autonomous drone needing to navigate a collapsed mine, deployed to assess a rapidly changing environment. Edge AI can power the decision making to navigate autonomously in an environment like this where the data - in this case the actual shape and pathways available in the mine to get humans out of danger - suddenly changed.
Empowering the future of innovation
We don’t yet know all the possibilities of AI and what it will become weeks, months, or years from now. However, even the most simplistic-seeming use cases of Edge AI can be powerful. The goal of a solution like NTT DATA’s is to empower third party developers by providing them with the tools to innovate, experiment, and come up with their own innovative use cases. It’s Shahid’s hope that we will someday see a democratization of Edge AI where end users, developers, and leaders across these non-IT world businesses can collaborate and share knowledge. Through technology, it becomes possible to empower workers with tools that can increase their utility, skill sets, and value.
As always, don’t forget to subscribe to Catalyst wherever you get your podcasts. We release a new episode every Tuesday, jam-packed with expert advice and actionable insights for creating digital experiences that move millions.
Episode hosts and guests

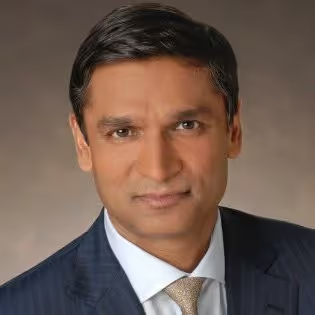
Written by
Catalyst Podcast
Episode transcript
Shahid Ahmed: Sometimes the sun goes around.
Clinton Bonner: (Laughs) It usually does. Right? Or something...
Shahid: Right.
Clinton: It's moving, right? Which is good, so.
(CATALYST INTRO MUSIC)
Clinton: Welcome to Catalyst, the Launch by NTT Data podcast. Catalyst is an ongoing discussion for digital leaders dissatisfied with the status quo, and yet optimistic about what's possible through smart technology and great people. Today, I'm sitting down with Shahid Ahmed, the Group EVP - that's executive vice president for those out there - of edge services at NTT Data. Shahid is a seasoned technology executive with a career spanning over two decades in driving digital transformation and innovation. He leads the charge at NTT Data in harnessing cutting-edge technologies to create digital experiences that have a big impact. His expertise ranges in IoT, private 5G, and our main topic today, the emerging world of edge AI. We couple that with his passion for leveraging smart technology to solve complex business challenges, and I think it's going to make for an incredible conversation. One last thing about Shahid: he's also so knowledgeable that he serves as an advisor to the FCC, which I think is pretty darn cool. So let's welcome to the studio for the very first time, Shahid Ahmed. Shahid, awesome to have you with us from Chicago. How are you doing today?
Shahid: I'm good, Clinton, thanks for having me part of your podcast. Looking forward to our conversation.
Clinton: When I was at our sales kickoff in Vegas, there was a couple of breakout sessions that really focused on edge AI and this combination of private 5G and what's now possible at the edge. And we got to meet a little bit, which was great. And I was so happy we could get you scheduled. Because for me, this topic is such a culmination of technologies that have been building, kind of, towards each other for a really long time, and I'm super excited to get into it with you. So I'll kick things right off, Shahid. We're saying a term that people might be like, well, I know what the two things are. I know what edge is. I know what AI is. But can you explain edge AI from your point of view? And also, if you could juxtapose that versus maybe traditional AI, I think that would be a great foundation to lay for the audience.
Shahid: Before I explain what edge AI is, maybe we take a quick step back and describe what has happening with AI, last two, three years?
Clinton: Yeah. Let's do it.
Shahid: Ever since ChatGPT came to the scene in September of 2022, things have changed. And they have changed mainly in the consumer context. So, you and I, we've been using AI in our daily lives, but also, we've seen AI take real shape within the enterprise and the IT side. And by and large, you know, even here at NTT, everybody, every one of our employees has Copilot. Much like many of the other larger Fortune 500 companies have implemented strategies where employees are taking advantage of Copilots, other AI assistants, to help them with their daily workloads, whether it's typing an email, summarizing a conference call, or, for that matter, reviewing a law, and legal papers, and being able to figure out what the next step might be. But all of that has been taking place in the, what I call IT world. No one's really paid attention to what's happening on the non-carpeted factory floors. The mining operations. The shipyards. Airports. Hospitals. When I speak to many of our customers, much like I did last three-four hours this morning...
Clinton: (Laughs)
Shahid: They're really struggling with implementing what has taken really strong foothold in the IT space, but not in the factory floor. And some of those workers and managers who manage the factory operations are like, how do we take advantage of AI in my factory floor? And that's kind of what our edge AI solution solves for. It's essentially the Aspirin for helping factory managers implement AI solutions in their really tough and rough environment, where they can't take advantage of AI in a meaningful way. So that's something we're very happy to launch. We just launched it a few weeks ago. We're seeing tremendous reaction from our customers, our partners, equally.
Clinton: Okay, so you got your factories, you have your hospitals. Where people are walking the physical floor, right? That's the thing we're talking about here. And that spans a lot of different industries right there, and in many, many ways. But what's possible when we're talking about edge AI now? You know, we know about, there's things out there. There's been computer vision for a long time. There's networks and keeping your data local and things of that nature. But when we're talking about implementing edge AI, what kind of benefits are we talking about to someone who, say, operates a hospital? Operates a really large manufacturing plant? What's now possible that simply just wasn't even 2 to 3 years ago while Copilot was coming online and being tinkered with, like you said, but it really hadn't bled out to the edge yet? So, what's now possible?
Shahid: So, let me give you a real life example we just implemented for one of our customers. Simple use case. A factory about... Over a million square feet. Big in terms of area and coverage of that factory. They have over 100 different thermostats in that factory. And one of the big problems this factory is trying to solve is energy consumption, to meet their sustainability goals and their net-zero ambitions. Energy consumption, as you know, it's top of mind for every manufacturing, discrete manufacturing or process manufacturing company. It's a big, not only opportunity, but objective for them. And so a simple use case they have is to have an ambient temperature of 72 degrees. And, you know, you and I have... probably have the same challenges in our homes where we've got multiple thermostats. We all want... It's just 72 degrees in our house, and we have to tinker around with a couple of thermostats, whether it's in the room that gets a lot of sunlight versus the room that does not get any sunlight, and we're trying to manage each one of them manually, right? Running around. Think of that example inside a factory floor. We've got hundreds of thermostats. Now, the problem statement is, how do you create a 72-degree ambient temperature across those, that huge square footage? What has happening today is that each one of those thermostats, while they has its own app, the operations manager has to look at all hundred of these thermostats discretely in his or her iPad. And tinker around with each one of them so it can reach 72 degrees. It's very painful, let me tell you that, because sometimes the sun goes around. You've got people opening doors, windows. There's heat emitted from the machineries itself. They may be thermostats and HVAC systems near very heavy mechanical area. So there's all kinds of dynamic things happening. And what we did was, took all of those hundred thermostats, and I'm oversimplifying it, but collected all that data into our edge AI platform, and then had a AI, simple AI algorithm, manage things actionably. Meaning it's an actionable AI. It dynamically sends commands down to each one of those thermostat apps and tells it to react accordingly in order to achieve 72 degrees. Very simple use case, but believe me, it's very difficult for them to achieve something like this. Because things change, are changing all the time, and you have to have a person almost dedicated to just managing all those hundreds of apps, and you still don't get it right. And so now you've got AI just doing this for you, sending commands to each one of those thermostats in a very quick, actionable way, and achieving that 72 degrees. It's a monumental lift, believe it or not, but it does tremendous things for the environment, energy consumption. It achieves the net-zero goals, sustainability goals for this company. Everybody wins.
Clinton: You know there's some beauty in that simplicity of that story, I think, Shahid. And for me, it's not just that the AI is out there gathering data and then saying, okay, there's an imbalance, in that particular case. Or maybe you have some computer vision AI out in factories, and it's noticing things on a factory floor are out of whack for whatever reason, right? Something fell. Something's in the way. It's not just saying, hey, there's a problem here. It is at the edge, actively computing, and then resolving. And in this case, getting to a homeostasis of 72 degrees in a million square foot place where, I can guarantee you, different parts of that floor are putting off, also putting off different heat signatures, because it's capital equipment, right? Which... So, all of that has to go into balance and be orchestrated in a really elegant way. And again, I love the simplicity of it, but I think it's a great achievement, and also a nice way to spotlight how you could think about it for other use cases, also. Like, so that's just one use case. And I'd love to understand, too, what's the culmination of technologies? Because I think that's an important piece of this as well. Yes, there's the edge AI platform, but what enables edge AI to even be edge AI and do its thing?
Shahid: Very simply, I call it actionable AI. So we're all used to gen AI, right?
Clinton: Yeah.
Shahid: You put in a query and say, tell me more about Clinton and where he resides in Connecticut as an example, right? And, or, I could be talking about Bill Clinton, right?
Clinton: Sure.
Shahid: And you can do that on your Gemini or Copilot or ChatGPT 4.0, what have you. Right? So perplexity is another one. That's understood. But what about AI taking real-time action on the data that it is receiving in real time? That's the transformation here. That's the difference between gen AI, LLM models, versus a actionable AI where it's dynamically learning. Go back to that thermostat example. It's learning that one of the machinery is emitting a lot of heat, so I need to actually cool it down, not do anything else. But other areas can remain the same. It's a linear programming model problem, right? Where you have a bunch of variables and you're trying to optimize for a single ambient temperature. But you're taking action on it in real time. That's the difference between your traditional LLM model, where it's very static, it's heuristic-based, statistical probabilistic model where you can tell, what's the next word that will come behind that. Versus a dynamic model where you don't know what is happening, and the model has to learn by itself. But you keep it very simple. Under 20 parameters, by the way.
Clinton: Right.
Shahid: So that it can run locally on that compute platform. You don't have to rely on an expensive GPU from Nvidia or anybody else. You can run it, in fact, actually on a Raspberry Pi-type small compute footprint, which is what we are affording to our customers, is a very small compute platform, but has the whole AI life cycle. Everything from AI editor to AI tester to AI storage module where you can store that small foundational model for that specific time series learning model for that algorithm. And so, another example I'll give you, and this is one of my favorite ones. We haven't rolled this out yet, but... If you can imagine a search and rescue drone. Where you send a drone into, I'm just making this up, in a cave or a mining operation where you don't know what's inside, right?
Clinton: Right.
Shahid: It's dark. There may be debris flying around. They may be things you haven't seen. And the drone itself has to be completely autopilot. Meaning, not even the video is working, but it's too dark. Or, you know, it's completely autonomous. It has to go from point A to point B and then back to point A. Safely, right? So it has to detect everything by itself. It has to learn everything on the go. And so, you can't rely on cloud. That's number one. To go back to some big algorithm. You can't rely on a big CPU because a drone is a drone has to be nimble and fast and small, by its very nature. And so, it has to make all those decisions, actionable decisions, in real time, near real time, right?
Clinton: Yep.
Shahid: Whether to move from debris or be able to navigate, cut corners, come back around and come back to its original position. But all of those actions have to take locally placed, I call it liquid AI, which is, it builds the neural network in real time. And that's the kind of methodology we are thinking about when we talk about factory context. Everything has to be in real time, much like that drone has to learn on the go. That factory algorithm has to learn on the go. Things go wrong.
Clinton: Constantly. And change, right? They're in flux. There is no... In the drone example, yes, there might be a map of the existing mine. In fact, I'm sure there is. But if there's a mine collapse, well, that map isn't as useful as it was 30 seconds ago, right? That things have changed on the ground. The idea, of course, is that the locality of it, the ability to, like you said, place things that are as small as or as less intrusive as, like, Raspberry Pi-style sizing, get the data you specifically need. That you think you're going to need, right? And you're measuring for, because you're probably thinking about future scenarios, or scenarios you want to help prevent, or get ahead of before there is a mining collapse in this example. And you're able to do that compute on the go, locally. And then, what about the data itself? Whether that's a factory or a hospital. You know, there's obviously data breaches, just data getting out to the public is, you know, it's like... It's every CIO, and then up to the CEO, and then up to the board's, worst nightmare, with data breaches and things getting out, whether that's from the cloud or on prem, but kind of the bigger versions of that. What's different at the edge?
Shahid: It's a big issue. I'm glad you brought that up, because a lot of people don't talk about this, and it is a key requirement. And we've seen this with CrowdStrike recently.
Clinton: Yeah.
Shahid: How exposed we are to some of these kinds of data challenges. And my perspective, and it's not just mine, it's all of our customers'... What they want, especially critical, mission critical data, that is directly responsible for their operations, inventory. Think of a product being built. They want that data to be local. You cannot have a CrowdStrike problem at Schneider Electric.
Clinton: Right.
Shahid: At BMW. Or a large electronics manufacturing company, or chemical, pharmaceutical company. Can you imagine if their systems were down, much like Delta had with their flight operations for, you know, not only days or weeks on? Even the Delta, I think the number was thrown around, was like a... At least a $500 million problem. In just the week, right? Lost revenue, cost to fix and all of that. So, this is something these factories take very seriously. They want to keep all their data local for that reason, not only because, for security risks and threats. They want to do it because they feel like this data is so critical that any process improvements, efficiencies, automations that you want to implement, it has to be locally implemented and managed. So data stays local. And in fact, even within our edge-AI architecture, we have built a airtight system for data. It never leaves. It never crosses the line to cloud infrastructure. Everything stays local,
Clinton: That unto itself is a bit of a platform, or at least a platform booster. Because now that it can stay local, because of all the advancements you're talking about, then you could start to do the compute and bring in those edge AI decision making abilities right there, and know you're doing it securely. Which is... It's almost a non-starter, right? If that, if it... First of all, it can't go to the cloud and come back, and in time, there's a real actual physics problem there, to go to where it's got to go to, come back and compute with as low latency as humanly possible and as technologically possible. And then, of course, if it were to go out to the cloud, then you were exposing some data you just clearly just don't want out there. So that is an enabler of empowering this next wave of the use of AI, which again, on the edge, we're calling it. So, how about for technology leaders that, you know, whether they're hearing this now or they're at conferences and they're getting around these concepts of how they might be able to apply edge AI. When you're walking factories and you're talking with those CIOs, CTOs and CEOs who are like, yes, we want to modernize this and we believe in the promise of edge AI. Where are they seeing most of their technology challenges? That could be physical or technical? How do you guide them? What are the things that kind of come up over and over again when they are trying to lean into this?
Shahid: Well, one of the things, and I mentioned this earlier, is that every CIO in, at least in the manufacturing industrial sector, and I include, you know, mining operations, shipyards, airports, all bundled into that same vertical. You know, one of the big queries that we get from them is, how we can take advantage of AI for my manufacturing plant?
Clinton: Right.
Shahid: In my factory floor. That's one of the key things that I hear a lot, right? They see what's happening in our own daily lives. They see what's happening in the IT world, and they want to be part of that bandwagon. And it's a double-edged sword, however. They recognize that. Because they know the power of AI also means that that data will end up somewhere else in the cloud. So, they have to manage that very carefully. And so that's why they like this idea of edge AI, where everything kind of happens locally. It solves their problems, right? You know, it's not about AI... And Steve Jobs very clearly articulated that 15, 20 years ago, where he said, you know, let's not solve for technology, it's great. Let's solve for customer experience. What is the experience you're trying to instill? What's the end experience, user experience you're trying to achieve? And then work backwards. And to me, that's one of our critical philosophical principles that we implemented as part of edge AI architecture, which is, let's solve for what is the customer looking for? All the way from, how do they build interesting, useful, creative AI use cases? And so we created the AI editor for them. And then, how do they want the data to be presented in a manner that AI could use it? So we created a data plane where we ingested all the data, cleaned it up, structured it, and put it in a nice, easily consumable way for the AI developer, who knows really well AI, has written a bunch of Ruby algorithms and code, but doesn't know how, you know, manufacturing data is structured. So, we did that for them. Then we created testers. Then we created evaluators. Making sure that's the experience they're looking for. You know, we kind of solved for what the end user was looking for. And really, in our mind, end user is the developer for the factory operations, and the factory manager himself or herself, which is to make sure they're achieving their, whatever, KPIs, whether it's sustainability goals, safety goals and others. So... That was sort of our mindset when we were thinking about this.
Clinton: I think it's well thought out. And it's covering different areas, like you said. Even, like, the AI editor, and I'd love to dive into that next, and the AI editor is, again, another enabler that allows the developer, locally, to prompt and play. And really tinker, right? And try to understand what's possible in these almost, like, micro-experiments, which I think is really, really interesting. Because that's how you get a lot of... Well, you get experimentation, so you get a lot of trial at a low dollar, or very, very low dollar swing. Just maybe the time it took to create the next prompts and fire off a little experiment to see, well, what can we do here? Will this either solve a problem, or can this provide some new value that we weren't even thinking about yet? And when you have a platform like that and you're enabling... You're kind of dollar cost averaging the swings you could take on it, because all the infrastructure is there, you really have a recipe for innovation. And for growth. Because you have enough... You're allowing for experimentation. You know, in the use case of the 72 degree homeostasis for the huge factory. Okay, cool. That's a great example. And it sounds like the client knew what they wanted to do. We have a sustainability goal. We understand already, because we already have a human trying to be the greatest maestro of all time and tinker a hundred different, you know, thermostats in real time...
Shahid: Like a DJ!
Clinton: Yeah, exactly, exactly. And hey...
Shahid: Literally, they had the knobs, and I looked at it, I'm like... You've got hundreds of these. You still need to scroll over to the next screen to see the other...
Clinton: (Laughs) Right. It's almost like a typewriter, too, because by the time you get to the end screen, you've got to, you know, shove it back to the beginning and see what's changed since you just did it. But in that case, they knew the use case. I would imagine there's lots of people out there - again, CTOs, CIOs that are walking the floor - that don't know yet what they could do. It's like, okay, this is new. You know, it's a new combination of technologies. How do you guide clients in that place. Which I would even guess might even be more the majority than the minority, or certainly a healthy chunk, that are like, I understand, like you said, I need to get on the bandwagon and use this, but I don't really know the use cases yet. How do you go about finding and then defining and then saying, yes, we have a few that we believe could drive a lot of value. What's that process like?
Shahid: You know, look. I always tell my team, too, we don't know all the possible AI use cases.
Clinton: Yeah, sure.
Shahid: I mean, this thermostat, even though it's very simple, it's very powerful. In that it captures the essence of what the art of the possibility is. That's just one. I don't know what might be out there today, but what I know, what we can do, is we can build the platform where third-party developers can innovate and experiment, even try out different ideas to come up with a beautiful use case. Let's give them the tools.
Clinton: Mm.
Shahid: Let's give them the data. Protected, secure. Locally managed and executed. Give them that facility. It's almost going back to the old adage, I wanted to not be... In the gold rush, I didn't want to be the guy digging for gold, but I wanted to be the guy who created the spades and the Levi jeans.
Clinton: Yep.
Shahid: I really wanted to give them the right tools.
Clinton: Yeah, for sure.
Shahid: Yes, we want this to be a profitable business. But really, that is the big challenge, is making available the right tools for the right type of developers inside the factory. That's our target segment and user base. We want to make sure not only the end user customer, the factory manager, but really, the developers are taking advantage. And doing what they do well.
Clinton: Right.
Shahid: Is try out different things. They might come up with something, you know, you and I have not heard of. But let's give them the tools.
Clinton: I'm sure they will. I'm certain they will. Because that's just the way it has happened throughout history when you create a, like, a neo-platform, which is what this is, and then you let smart people who are curious and skilled enough to go at it. And the cool part about AI in general, too, is that there is an innate democratization of it, because it might not be hardcore coding to at least start some of the things to experiment. It's just learning a language of prompting properly. And you mentioned the AI editor, and in fact, I don't think I have to ask you about it more because you wove it into the last answer really nicely, it's like, hey, it's a part of a tool set that enables creativity, experimentation. And getting the whole thing set up from the infrastructure to the toolkit to experiment. And, I'm assuming, probably some of the consulting, and maybe some of the change management that goes around. Things physically change, like when you put these solutions in a physical space, not only are new things doable, but now new teams might be coming together, also. Like, brand new teams that hadn't worked, you know, yet together. That, all of a sudden they're in the same boat, if you will. And they could collaborate in a whole new way, which is really an interesting piece as well. So, I always find that kind of inflection point fascinating. And I'm seeing it a lot currently. A different field where we're seeing that a lot, in my opinion, is in VR. But then the specialty applications within VR that the barrier to entry to go play, if you will, is becoming so, so low. So, like, real time digital twins where just the... Anybody could get in and walk around and annotate and collaborate in a new virtual twin space. It feels similar to me that we're going to have this beautiful melting pot of, let's call them, traditional people that are operating the factory floor, and the folks who really understand the operations of the physicality, and then the next-gen folks who could come in and really amazingly uplevel the efficiencies in productivity, or like you said, safety measures within. So, just a brilliant, I think, culmination. So, one last question for you would be, what do you believe is next, right? So this is pretty new as it is. And the pace is very, very, very fast. So we have private 5G. We have this emergence of edge AI. You're seeing these use cases be born. What do you think is even the next wave after this, or a progression that really takes this and makes it a mainstream thing, where every major company that operates buildings and facilities and physical spaces like this, that they are rushing to this because they see such advantages?
Shahid: I like the way you framed it, the democratization of AI. I see that same thing in the manufacturing, mining operations, oil rigs. Code development, code generation is one of the biggest use cases for AI. And so, I'd love to see a GitHub-type environment for the manufacturing sector. Right?
Clinton: That would be amazing.
Shahid: Right?
Clinton: The uptake that you can get there, right? It's not just standardization, but you just keep getting contribution, and then constant upleveling of what's possible, because you have so many contributors. And then you could lean into what people have done before you very quickly and spin up solutions. Yeah.
Shahid: Correct. It expands, like, you know, the factory, or that rig worker in that offshore rig in southern Atlantic. You know, you've got somebody who's probably trying to upscale themselves, and now is able to just get their laptop out, connect to the edge AI platform, have the AI editor generate code for them, whether it's Ruby on Rails or any other language that edge AI platform uses, but do it in a very meaningful way in that editor. And have all the data in there. And be able to do, solve their problem. Right? And then that person goes back home to South Carolina, east coast of US, and is able to apply that knowledge elsewhere. You're really powering, empowering that worker, that otherwise we call blue-collar worker, with tools that have really increased their utility, their skill set, their value across the board. I think, to me, that's the most powerful thing we can do for our factory workers, our people who wake up every morning, roll up their sleeves, go to these warehouses and really improve the GDP of the whole... Not just one country, but the whole world.
Clinton: Yeah, I think that's an awesome and inspiring place to end it, Shahid, so... Thank you so much for joining us today and diving deep into the transformative world of edge AI. I feel like we've covered a lot, from understanding how edge AI differs from traditional AI, and the consumerism of it, as we talked about, over to exploring the key benefits across myriad industries, and some great use cases you shared, too. And then also, tackling the technical challenges and the innovative solutions that come with a burgeoning platform. So, your insights into the real world applications and the promising trends for the future have been really incredibly enlightening. It's clear to me that your expertise and your passion for leveraging the smart tech to really change things for the better, it shines through and really, really, truly stands out. So thank you again for joining us on the podcast.
Shahid: Thank you, Clinton. And I'm going to try to get that cool mic and headphones for our next podcast.
Clinton: (Laughs) You don't have to be a podcast host to get one. You can just go on Amazon. Talk about democratization, right? You get that podcast are a great democratization story. Anybody can have a voice. Well, for the listeners out there that are enjoying the Catalyst conversations, please share the podcast with colleagues and friends. Because in this studio, we believe in shipping software over slideware, that fast will follow smooth, and aiming to create digital experiences that move millions is a very worthy pursuit. Join us next time as the pursuit continues on Catalyst, the Launch by NTT Data podcast.
(CATALYST OUTRO MUSIC)